Category Archives: Uncategorized
Cardiac Arrhythmia Classification by Multi-Layer Perceptron and Convolution Neural Networks
Cardiac Arrhythmia Classification by Multi-Layer Perceptron and Convolution Neural Networks
Shalin Savalia 1,* and Vahid Emamian 2
PUBLICATIONS IN MACHINE LEARNING, DEEP LEARNING, AND NEURAL NETWORKS
DR. EMAMIAN’S PUBLICATIONS IN MACHINE LEARNING, DEEP LEARNING, AND NEURAL NETWORKS (2000-PRESENT):
- N. Ghebremeskel, V. Emamian, “Application of Convolutional Neural Network and Deep Learning for Detection of Cardiac Arrhythmia Heart Disease”, Published in Journal of Research and Advancement in Electrical Engineering, Volume 3 Issue 1, 2020.
- N. Ghebremeskel, V. Emamian, “Classification of Cardiac Arrhythmia Using a 2 d Convolutional Neural Network”, Int Journal of Scientific Research, 10(09), pp. 35620-35625, 2019
- N. Ghebremeskel, V. Emamian , “ECG Arrhythmia Classification Using a Convolution Neural Network”, Engineering and Technology Journal, Vol 4 No 04 (2019): Volume 04, 30 April 2019, Page No.: 570-577
- M. Rane, K. Shah, V. Emamian, “Blood Pressure Estimation Using Electrocardiogram and Photoplethysmogram”, Published in International Journal of Science and Engineering, Page No. 20 to 25, December 2018.
- K. Shah, M. Rane, V. Emamian, “Detection of Heart Defects using Electrocardiogram (ECG)”, Published in International Journal of Science and Engineering, Page No. 15 to 19, November 2018.
- S. Savalia, V. Emamian, “Cardiac Arrhythmia Classification by Multi-LayerPerceptron and Convolution Neural Networks”, Published in MDPI Journal of Bioengineering, May 4, 2018.
- A. Arivalagan, N. N. Peddi, W. Bazuhair,V. Emamian, “Study of Wireless Security Attacks on Medical Devices”, Published in Journal of Multidisciplinary in Cryptology and Information Security, ISSN 2320 -2610, Volume 6, No.6, December 2017.
- S. Savalia, E. Acosta, V. Emamian, “Classification of Cardiovascular Disease Using Feature Extraction and Artificial Neural Networks”, Published in Journal of Biosciences and Medicines, ISSN Print: 2327-5081, Pages 64-79, Nov 2017.
- S. Alomari, M. Shujauddin, V. Emamian, “EKG Signals – De-noising and Features Extraction”, Published in American Journal of Biomedical Engineering ISSN: 2163-1077, pp 180-201, 2016.
- A. R. J. Fredo.,R. S. Abilash, V. Emamian, “Segmentation and analysis of cortical, subcortical and subanatomical regions in autistic MR brain images using local and global energy based multiphase level sets”, Published in journal of Computer Methods in Biomechanics and Biomedical Engineering, 2016.
- A. Alharthi. V. Emamian, “Automated Mechanism for Early Screening and Diagnosis of Diabetic Retinopathy in Human Retinal Images.” published in British Journal of Applied Science & Technology, Volume 11 Issue 6, 2015.
- S. Shahhosseini, B. Rezaie, V. Emamian, “Sequential Image Registration for Astronomical Images”, ISM, 2012, 2013 IEEE International Symposium on Multimedia, 2013 IEEE International Symposium on Multimedia 2012, pp. 314-317, doi:10.1109/ISM.2012.65
- A. Alharthi. V. Emamian, “Application of Smartphone for Early Screening and Diagnosis of Diabetic Retinopathy”, Published in Journal of Diabetes Mellitus, Volume 4, No 4, Oct 2015.
- S. Shahhosseini, B. Rezaie, V. Emamian, “Sequential Image Registration for Astronomical Images”, Submitted to the 2012 ISM (The IEEE International Symposium on Multimedia)
- C. Sauer, V. Emamian, “Application of Guided Wave Technology and DSP Techniques for Fault Detection and Characterization”, Appeared in IEEE International Conference on System of Systems Engineering, April 16-18, 2007, San Antonio, Texas.
- C. Sauer, V. Emamian, “Fault Detection and characterization using Guided Wave Technology and Signal Processing Techniques”, ISSPIT 2006, The 6th IEEE International Symposium on Signal Processing and Information Technology, August 27-30, 2006, Vancouver, Canada
- V. Emamian, M. Kaveh, A. H. Tewfik, Z. Shi, L. Jacobs, and J. Jarzynski, “Robust Clustering of Acoustic Emission Signals Using Neural Networks and Signal Subspace Projections”, Published in Journal on Applied Signal Processing, March 2003 issue.
- V. Emamian, M. Kaveh, A. H. Tewfik, and Z. Shi, “Acoustic Emission Classification Using Signal Subspace Projection”, Published in Proceedings of IEEE International Conference on Acoustics, Speech, and Signal Processing 2001, vol. 5, Salt Lake City, Utah, May 2001.
- V. Emamian, M. Kaveh, and A. H. Tewfik, “Acoustic Emission Classification for Failure Prediction Due to Mechanical Fatigue”, Published in Proceeding of SPIE conference on Sensory Phenomena and Measurement Instrumentation for Smart Structure and Materials, March 2000.
- V. Emamian, M. Kaveh, and A. H. Tewfik, “Robust Clustering of Acoustic Emission Signals Using the Kohonen Network”, Published in Proceedings of IEEE International Conference on Acoustics, Speech, and Signal Processing 2000, pp 3891-3894, vol.6, Istanbul, Turkey, June 2000.
Deep Learning, Machine Learning, and AI
Question: Are AI (Artificial intelligence), Machine Learning, and Deep Learning the same concepts?
Deep learning and machine learning are ways of achieving AI. They are considered by many to be the same concept, but in fact they are different. Below, we try to explain the differences:
AI (Artificial Intelligence): AI is not the same as Machine learning or deep learning. Deep learning and machine learning both are considered the subsets of AI. Generally, AI is a computer program that is able to act similar to a human brain, in a way the brain works, thinks, and functions.
Machine Learning: Machine learning is somehow similar to data mining, which is a technique of examining a large database and extracting new information. Machine Learning is a technique of learning from that data and then apply what we have learned to make an informed decision. Many companies use machine learning to give their customers a better experience, such as Netflix who uses machine learning to give smart suggestions to their customers of movies or TV shows that they would like to watch, or Amazon who uses machine learning to give product recommendations based on their preferences.
Deep Learning: Deep learning is a subset of machine learning. The difference is the number of hidden layers used in deep learning is much more than machine learning. If a machine learning model ends up with an inaccurate prediction then the program or model needs to be fixed or improved, however, in the case of deep learning, the model does it by himself. Self-driving car is a good example of deep learning.
Machine Learning Research
University gets $268K grant to enhance deep learning
May 28, 2019
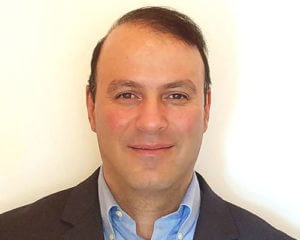
A $268,000 grant from the U.S. Army Research Office, via the Defense University Research Instrumentation Program, will help St. Mary’s University purchase cutting-edge computer equipment for improved research in deep neural networks (deep learning) and artificial intelligence (AI).
According to Professor of Electrical Engineering Vahid Emamian, Ph.D., the grant’s principal investigator, the money will buy a deep learning platform from a company called AMAX.
Deep learning is a subset of machine learning in which artificial neural networks — algorithms inspired by the human brain — learn from large amounts of data.
“My research involves various aspects of deep learning and AI in automatic health monitoring as well as signal and image processing,” Emamian said. “For example, in health monitoring, I can leverage AI in predicting a patient’s future risk of stroke or heart attack, using continuous data collected from body sensors.”
Emamian said the new equipment “exponentially increases the processing power, enabling me to run more complex simulations.”
Engineering, Computer Science, Mathematics and Physics faculty will be able to incorporate the equipment into their teaching and research.
Part of the goal is to attract current and prospective St. Mary’s students to careers in science and engineering, Emamian said.
“Developing effective instrument-based education will impact not only hundreds of St. Mary’s students annually, but also a select audience from other U.S. universities or institutions as students will be able to securely access the platform over the internet to run AI and deep learning simulations off campus,” he said.